Introduction:
POCs, or proof of concepts, are recurring challenges in the trajectory of SciCrop.
For us, they are always great opportunities to address the pain points of our clients through the presentation of concrete solutions based on big data analytics, in this case, through our on-demand algorithms service.
In recent months, we have been working on the proposition of machine learning-based climate monitoring and forecasting models to optimize planting in large tobacco farms in the southern region of Brazil.
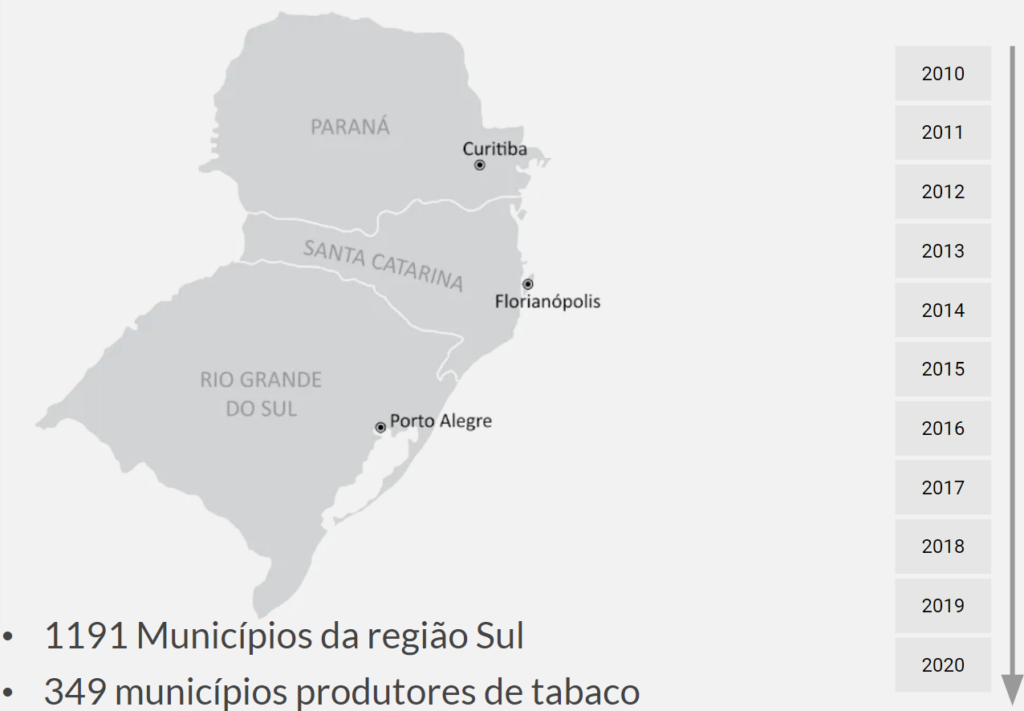
The Challenge:
The challenge was to determine the reliability of the current rainfall forecasting model used and propose long-term improvements.
In all crops, regardless of the genus, climate is the most impacting factor in terms of productivity, and for tobacco, it is no different.
Especially when it comes to tobacco, gaining predictability about rainfall conditions is essential for planning management operations, since the species depends on regular rains to reach its full development.
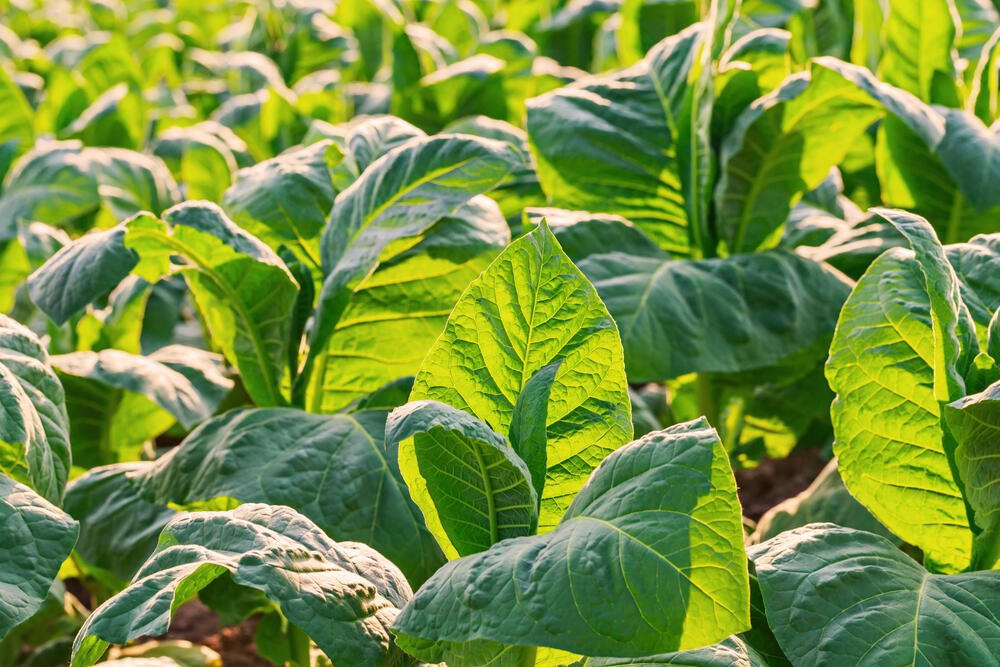
In an attempt to obtain this foresight, the client relied on a “traditional” forecasting solution that did not correspond satisfactorily to the incidence of rainfall.
It was necessary to evaluate the accuracy of the current model and work on proposals to achieve a higher degree of confidence in the future.
The Solution:
Using machine learning and historical rainfall data from more than 10 municipalities, dating from 2010-2017, our model established predictions of mm of rain in the next 30, 60, 90 days of the following years.
Based on these forecasts, we conducted an accuracy comparison between our model, the model used by the client, and the actual rainfall indexes.
The results showed a great disparity in terms of accuracy, with the SciCrop model being approximately three times more assertive than the one contracted by the client.
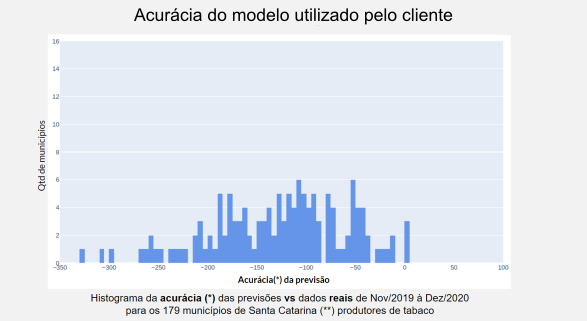
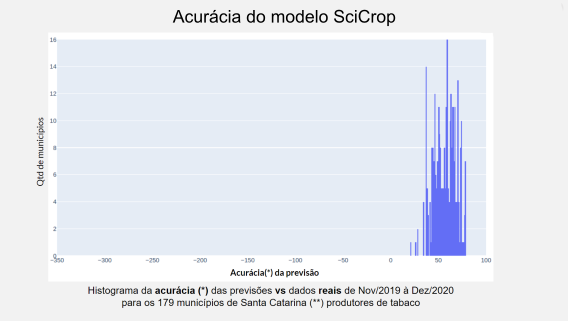
The Result:
Using its analytics expertise, SciCrop presented, based on statistical indicators, the low reliability of the model in use, delivering a precise diagnosis of the problem faced by the client.
In this POC, we went beyond the finding: we showed the client the concept of hyper-localized climate forecasts and how the application of advanced analytics can outperform one of the leading traditional rainfall forecasting models on the market.
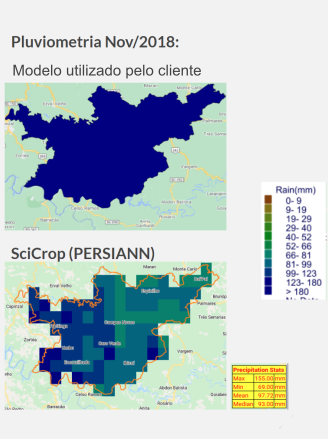
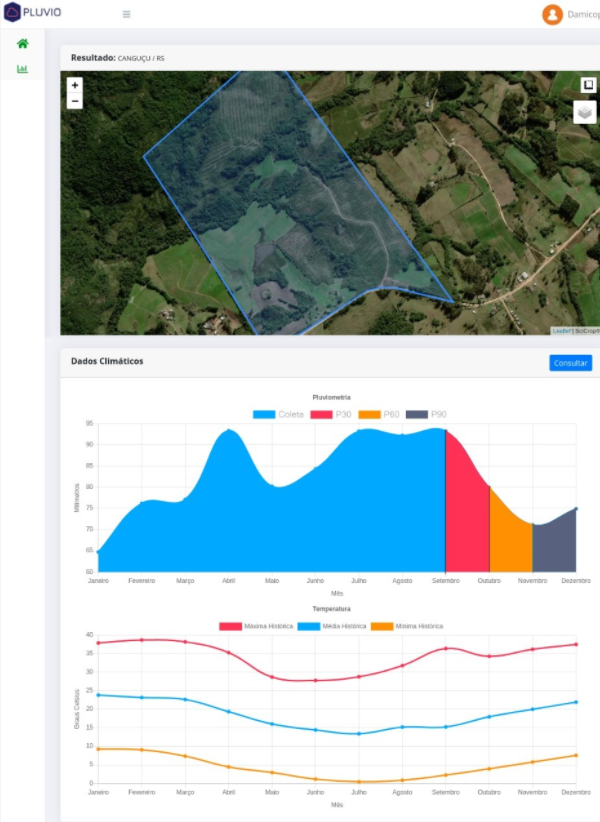
Conclusion:
Don’t let your agricultural teams make decisions based on dubious information.
Contact SciCrop and start counting on the latest data analysis technologies to guide strategic actions in your rural property or company.
Call to action: